How to Optimize Your Dedicated Server for Real-Time Analytics Applications
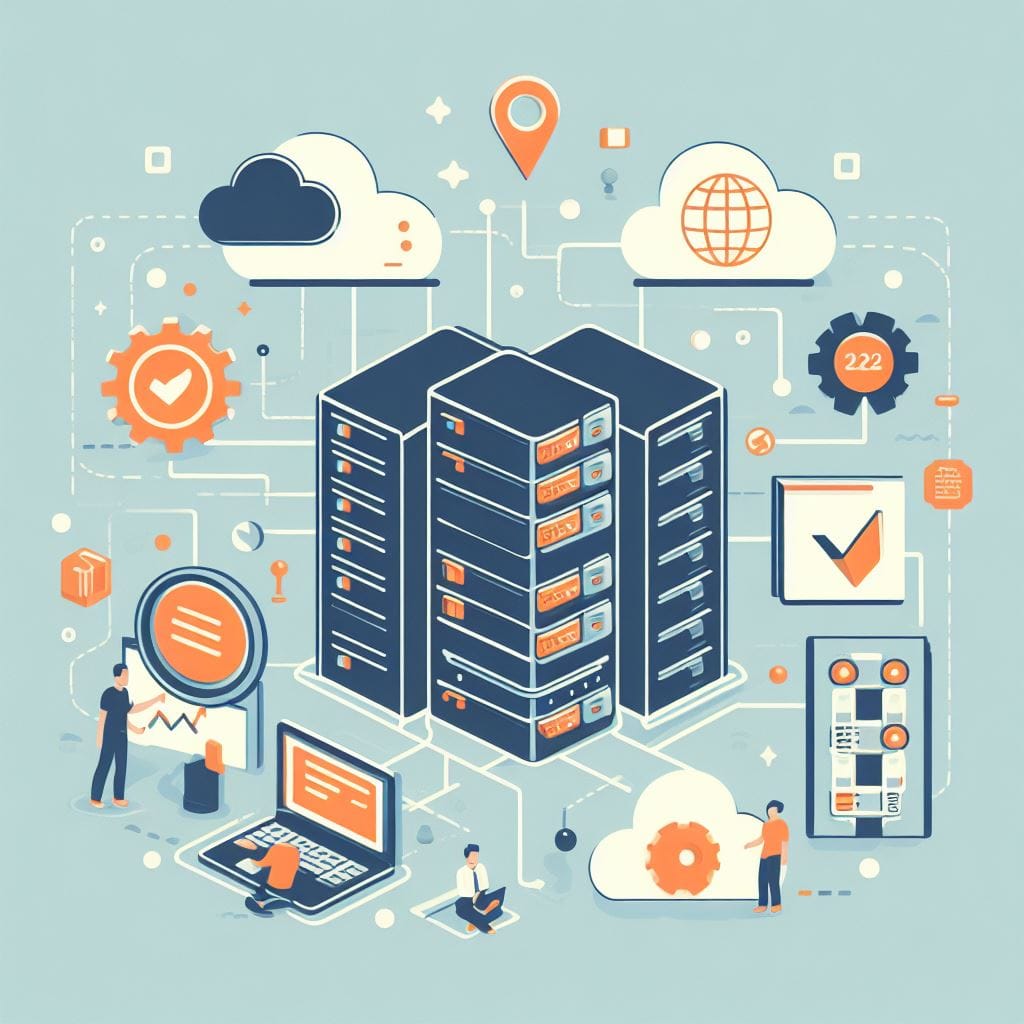
Optimizing a dedicated server for real-time analytics applications involves several steps to ensure that the server performs efficiently and reliably. Here are some key strategies you can employ:
- Selecting Hardware:
- CPU: Choose a high-performance multicore processor. Real-time analytics benefit from processors with higher clock speeds and multiple cores.
- RAM: Opt for a large amount of RAM. The more RAM available, the better the server can handle concurrent analytics queries.
- Storage: Use SSDs (Solid State Drives) for faster read/write operations. SSDs can significantly speed up data retrieval.
- Network: A high-bandwidth, low-latency network interface is crucial for real-time analytics.
- Operating System and File System:
- Choose a lightweight and stable operating system. Linux distributions like CentOS, Ubuntu Server, or a specialized distribution like CentOS Atomic Host can be good choices.
- Utilize a file system optimized for performance, such as ext4 or XFS on Linux.
- Database Optimization:
- If you're using a database, ensure it's properly configured and indexed for fast queries. In-memory databases like Redis or Memcached can be particularly effective for real-time applications.
- Parallel Processing:
- Configure the server and applications to utilize parallel processing whenever possible. This helps distribute the workload across multiple CPU cores.
- Load Balancing:
- Implement load balancing to distribute incoming requests evenly across multiple servers. This can help prevent any single server from becoming a bottleneck.
- Caching:
- Utilize caching mechanisms to store frequently accessed data in memory. This reduces the need for repeated disk I/O operations, significantly improving performance.
- Data Compression and Serialization:
- Use efficient data compression techniques to reduce the amount of data transferred over the network. Additionally, consider using lightweight serialization formats like Protocol Buffers or MessagePack.
- Monitoring and Optimization Tools:
- Implement monitoring tools to keep track of server performance. Tools like Prometheus, Grafana, and New Relic can provide valuable insights into server health and performance.
- Security Measures:
- Implement security best practices to safeguard your server and data. This includes firewalls, regular security audits, and timely application of security patches.
- Optimize Queries and Code:
- Optimize the queries and code of your real-time analytics applications. Ensure that queries are well-structured, and indexes are used effectively. Use efficient algorithms and data structures.
- Regular Maintenance and Updates:
- Regularly update the server's software, including the operating system, database, and application stack, to benefit from performance improvements and security patches.
- Backup and Redundancy:
- Implement a robust backup and redundancy strategy to ensure data integrity and availability in case of failures.
- Scale Horizontally or Vertically:
- Depending on your specific needs, consider scaling your server resources either by upgrading to a more powerful machine (vertical scaling) or by adding more servers (horizontal scaling).
Remember that optimizing a server for real-time analytics is an ongoing process. Regular monitoring, testing, and tweaking based on actual usage patterns will help maintain optimal performance over time. Additionally, consider consulting with a system administrator or DevOps engineer for specific guidance based on your application and server setup.